Interview with Mike Bowles, Head of Quantitative Finance at Collaborative Drug Discovery (CDD), Inc. PharmaKB Project
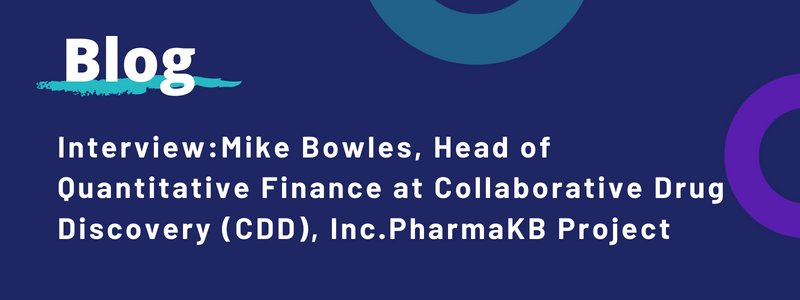
Please give us a little introduction on your current role and what you do
I'm currently the Head of Quantitative Finance at CDD, where we are exploring new information sources for investors in pharmaceuticals and drug companies. I have a background in Quantitative Finance and trading, and specialise in Machine Learning, providing consulting services across a variety of industries, from finance to healthcare and manufacturing. In addition to this, I teach Machine Learning at a local university and have a keen interest in the use of Machine Learning to solve financial and economic problems. I am passionate about equipping people with the knowledge and tools to make informed decisions based on data.
What have been your /your firm’s top 3 priorities for the coming year?
Collaborative Drug Discovery (CDD) believes semantically integrating pharmaceutical industry data provides an edge to investors. We’re semantically integrating numerous disparate data sets so investors can forecast the near-term and long-term performance of drugs and the companies that produce them.
Over the past 2 years CDD has constructed the PharmaKB system to capitalise upon this opportunity.
Our priorities for this year are as follows:
1) Complete the semantic integration of public data on Companies, Drugs, and the Diseases for all approved commercial drugs and drugs in clinical trials.
2) Develop a pool of PharmaKB early adopter financial investors to help guide the next phase of development of PharmaKB
3) Expand our custom data portal to include a range of useful time-series indexed data sets to facilitate back-testing of quantitative investment strategies.
Don't miss new reports! Sign up for Quant Strats Newsletter
What do you think are the biggest challenges facing data scientists/AI experts/quantitative practitioners in 2023 and beyond?
Dealing with interest rate changes and risks to GDP growth are big challenges. It’s useful to note that worldwide yearly pharmaceutical revenues have increased every single year since 2000. (according to Statistica).
Market and political uncertainty over the last year has seen unpredictable outcomes for some quant firms – how do you think quant firms can prepare for increased uncertainty to come and manage the 40-year inflation high that was seen in 2022?
Quant firms can prepare for increased uncertainty by diversifying their portfolios and strategies, employing hedging techniques such as options and futures, and monitoring market trends and political developments closely. Additionally, they should focus on investments with low-risk and high-returns and consider tactical asset allocation strategies to help manage inflation.
To what extent do you see the use of blockchain/crypto integrating into capital markets? As crypto is becoming more mainstream, how have hedge funds responded and what could be the potential impact on capital markets?
a. What are your predictions for quant investing in crypto?
As long as the public is willing to speculate, there will be a market and market makers. The world-record frauds have probably dampened things for a while.
What is your advice to funds hoping to get new systematic strategies into production quickly and more often?
The key to systematic strategies is to hypothesise and backtest rapidly. To get new systematic strategies into production quickly and more often, it is important to have access to high quality data. Given the revenue stability of drug revenues, they are a good starting point.
ESG and sustainable investing is still a key topic but something that quant professionals are not always engaged with. How do you see this progressing in the coming years and influencing portfolio management?
In the coming years, ESG and sustainable investing will play a larger role in portfolio management. As sustainability issues become increasingly important to investors, quant professionals will need to consider ESG factors when making decisions about portfolio allocations, risk management, and other investment strategies. As ESG and sustainable investing become more prevalent, quant professionals will need to develop the skills and techniques to properly incorporate these considerations into their decision-making processes.
Privacy and regulation surrounding the responsibility and ownership of data is still an area being discussed. What measures are you predicting will be put in place to navigate any foreseeable data privacy challenges while searching for alpha, and how can funds learn to navigate these regulations and policies?
Predicted measures to navigate foreseeable data privacy challenges while searching for alpha include implementing strong data governance policies, developing and utilizing data-protection protocols, and ensuring compliance with relevant regulations and laws. Funds should ensure that they are familiar with the relevant regulations and policies, and consider investing in technology to help them comply with such regulations and policies.
To get a flavour of the Quant Strats full program download the agenda here.
Alternative data is still considered a source of alpha for many – what roadblocks do firms tend to come across in sourcing, cleaning, and using this data? How do you view the alt data market at present?
The biggest roadblocks firms tend to come across when sourcing, cleaning, and using alternative data are data availability and access, data accuracy and quality, and data integration with existing systems. The alt data market is growing rapidly, with more and more firms seeing the value in leveraging alternative data to gain a competitive edge.
Our research this year showed a lot more firms and practitioners talking about NLP than usual – why do you think this is? Where are you seeing the optimal utility for NLP and where does it have the potential to go?
I think there is a lot of excitement surrounding NLP because of its potential to automate and improve many tasks that were previously done manually or not at all. With advances in computational power and machine learning, NLP is becoming increasingly accessible and powerful.
In the future, NLP has the potential to be used in a wide range of applications such as automated customer service, intelligent search, automated summarization, and text-based recommendation systems. NLP could also be used to automate the analysis of large datasets, allowing us to uncover meaningful insights from the data more efficiently and accurately than ever before. These and other applications will spawn new companies and new approaches to business in existing ones.
Don't miss your chance to hear from 50+ speakers at Quant Strats on March 14, register here today!