Speaker Interview: Morgan Slade, Founder and CEO at CloudQuant
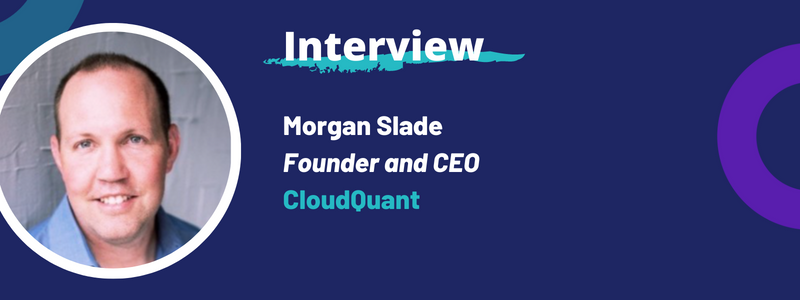
Please give us a little introduction on your current role and what you do
Founder and CEO of CloudQuant, a one-stop shop for all types of data, research insights, investment signals, and a full tech stack of solutions for coders and non-tech savvy users. I lead the research and sales efforts for CloudQuant.
What do you consider your biggest professional achievement to date?
Over the course of my career, I’ve had the opportunity to apply systematic strategies to every major asset class in both large sell-side and buy-side settings. I began my career as a trader for one of the largest and oldest systematic global-macro funds in the world and continued to trade at various points throughout my career at firms such as Citadel and Carlson capital. The most exciting thing I’ve done was to help turn around and take public a firm that ultimately became APEX Clearing. Connecting practical experience as an institutional trader with all the other quantitative and AI skills has been the most enjoyable and rewarding experience I’ve had.
What has been your /your firm’s top 3 priorities for the coming year?
● Identify the most interesting datasets for investing
● Develop the highest-octane signals from new datasets or approaches
● Make data and signals accessible to all institutional investors via Liberator API
What do you think are the biggest challenges facing data scientists/AI experts/quantitative practitioners in 2023 and beyond?
Open-source tools and educational resources have made AI accessible to a rapidly expanding cohort of data scientists and quants, however, without high-quality data there can be no progress toward improved prediction in markets. You either ask different questions of the same data or you obtain better data. The latter is an easier solution for most firms at this point. Our firm is focused on providing a firehose of real-time and historical data for AI-empowered researchers.
Don't miss new reports! Sign up for Quant Strats Newsletter
Market and political uncertainty over the last year has seen unpredictable outcomes for some quant firms – how do you think quant firms can prepare for increased uncertainty to come and manage the 40-year inflation high that was seen in 2022?
The Macro climate has gone through a regime shift that currently requires an awareness of how the economy works. In times of macro crisis, it is not uncommon to see historical statistical relationship break down for extended periods of time reflecting dramatic imbalances that dominate outcomes. The significant growth in M2 and Quantitative easing during COVID-19 resulted in significant distortions in the market. Understanding the source of stress is worth understanding to inform systematic investing. Traditionally firms have relied on government numbers to understand the macro environment. The most advanced firms are using real-time alternative data to understand the macro environment and are gaining an information advantage measured in weeks and months. This enables a greater interpretation of market risk, performance, and seemingly idiosyncratic behaviors that make sense in the context of the emerging macro picture.
To what extent do you see the use of blockchain/crypto integrating into capital markets? As crypto is becoming more mainstream, how have hedge funds responded and what could be the potential impact on capital markets?
a. What are your predictions for quant investing in crypto?
Crypto investing is a very inefficient market with side-car credit risk components when conversion back to fiat currencies is desired. Investor protection rules do not exist, so it is important to understand the types of behaviors that occur in unregulated markets. Innovations nonetheless serve a purpose and I believe the crypto experiment will yield beneficial technologies and assets in the long run. We predict that global assets will appreciate significantly later in the year. Crypto will become increasingly correlated with the credit cycle and appreciate at that time as well, albeit with higher volatility than traditional assets due to its speculative nature. Until crypto gains a bigger wallet share on the transaction side, it will continue to be driven by speculative spirits rather than fundamentals. We see a number of our hedge fund clients entering the space and requesting new crypto datasets to find an edge.
Speaking at Quant Strats
Morgan Slade, Founder and CEO at CloudQuant will be speaking at Quant Strats on March 14 participating on the panel taking place at 12.15 pm on the AI and ML Innovation track. The panel will be discussing how companies will be using machine learning to provide a true competitive advantage.
To get a flavour of Morgan's panel and the full program download the agenda here.
What is your advice to funds hoping to get new systematic strategies into production quickly and more often?
We suggest having a rock-solid foundation in the form of a point-in-time security master, price database, and backtesting engine. Without these standard tools, the research process will not be repeatable. Once you have that, then you will need novel datasets mapped on a point-in-time basis to tradeable securities. Those datasets need to be research-ready and bring a distinct perspective to the companies or assets in which you are investing. Machine learning can be a force multiplier for research especially if you have a data warehouse with the foundational fuel you will need to run the backtest engine.
ESG and sustainable investing is still a key topic but something that quant professionals are not always engaged with. How do you see this progressing in the coming years and influencing portfolio management?
Quants typically live and die by the performance. Fundamental managers can afford to be more philosophical about their outcomes, sometimes changing their investment thesis after a period of poor performance in hopes that something will change. Quants don’t have that luxury and it has been rare for ESG datasets to be positive return predictors. If that changes, you’d see every quant wishing for ESG data. We do have several ESG datasets we would recommend at CloudQuant for quants.
Privacy and regulation surrounding the responsibility and ownership of data is still an area being discussed. What measures are you predicting will be put in place to navigate any foreseeable data privacy challenges while searching for alpha, and how can funds learn to navigate these regulations and policies?
We utilize the FISD Due Diligence Questionnaire with our data vendors as part of our onboarding and vetting process at CloudQuant. We require an understanding of the privacy issues related to any dataset we analyze. Occasionally we are unable to clear the compliance hurdles necessary to accept datasets. By asking the right questions and conducting analysis of the data ourselves, in most cases we are able to ferret out likely issues before the data products are offered to clients via our Liberator Data Fabric. CloudQuant is a good filter for compliant, high-value datasets combined with a research team that can help you quickly understand a dataset.
Alternative data is still considered a source of alpha for many – what roadblocks do firms tend to come across in sourcing, cleaning, and using this data? How do you view the alt data market at present?
CloudQuant fills a gap for many in the capture of data on a Point-in-Time basis, the mapping of data to useful securities or entities, and the transformation of data formats into something easily queried and used in the institutional investing setting. We provide point-in-time capture services, data storage, entity mapping, and other curation services for many of our vendor clients to ensure that their data is research-ready and high quality. Normally firms will spend at least half their time duplicating this effort for themselves for untested, uncurated datasets. The cost of that process results in lower data trial velocity, slower innovation, and lower investor returns.
Our research this year showed a lot more firms and practitioners talking about NLP than usual – why do you think this is? Where are seeing the optimal utility for NLP and where does it have the potential to go?
There is little new science that predicts the spurts of progress in AI. The math underpinning deep learning has been understood for centuries, yet innovation has progressed at an accelerating pace. Progress inspires confidence which lights a fire behind human engagement and innovation. Sometimes that progress is predictable and boring, but occasionally something truly magical excites the human imagination. This results in more researchers putting in more hours with more clock cycles. The pace of progress proceeds nonlinearly with the nonlinear allocation of brainpower. There is also research supporting the idea that open-source collaboration is incredibly efficient for solving technical problems due to the collaborative nature and multidisciplinary approaches it encourages. Both of these key developments have created the Transformer models, drop-out, regularization, and a number of other architectures and engineering tricks that make Deep Learning models work. Expect this to continue. But remarkably, the rarest resource of all is clean, fresh, labeled training data. NLP models have gone mainstream and have been made accessible to the masses by leveraging the concept of transfer learning to create custom models in very little time and compute cost.
Don't miss your chance to hear from Morgan Slade, Founder and CEO at CloudQuant on March 14, register here today!