Interview: Suraj Gohil, Chief Strategy Officer and Co-founder, Fable Data
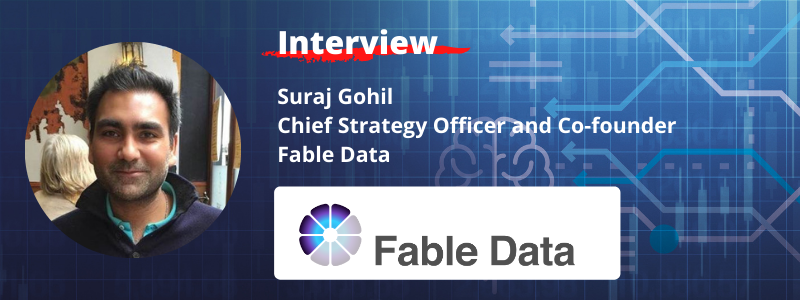
What do you think are the biggest challenges facing data scientists/AI experts/quantitative investors in 2021 post Covid-19 and why?
To say that Covid changed the way we are all living and spending would be an understatement. Most of the back testing carried out by funds, be it quantitative or fundamental, has been carried out using data and normalisation techniques from a pre-Covid environment. As Covid-19 broke many of these techniques, there was a scramble to recalibrate modelling. However, the issue with this recalibration was the lack of data points and, hence, validity. As the world emerges slowly from Covid-19, understanding which elements of modelling to take from during the Covid period and which to take from a pre-Covid environment will be the key to unlocking value – and this is not a trivial task. Many will rely on official data released by statistical agencies and governments, but these are often outdated and they lack the dynamism required to pivot in a constantly changing world. Will 2020 be seen as an aberration or a paradigm shift is the key question to answer.
Don't miss new reports! Sign up for The AI Data Science in Trading Newsletter
How do you see Biden’s presidency and policies on key issues affecting capital markets in 2021? What should funds and asset managers be focusing on?
Coronavirus: Controlling the pandemic and mitigating its accompanying economic fallout are the top priorities for the new administration. Biden’s policies have been much more inclined to follow the science-based approach to restrictions and lockdowns than prior government regimes. Arguably, this increases the risk of continued and increased volatility in consumer spend as we go forwards, if there are further lockdowns to come. With still much unknown about the effectiveness of the vaccine against further mutations, this will be key to watch. While we have seen subsequent waves of the virus be less volatile than the initial Mar/Apr/May 2020 wave of infections, this approach will arguably have an impact on key sectors as they re-open, including hospitality, travel and recreation. Alongside this, the administration appears to have a “whatever it takes” approach to stimulus, at least until the economic environment starts to improve. The impact of any deceleration of stimulus is arguably only going to be at a time when re-opening can support itself (in terms of economic growth). How this plays out will be key to consumer spending habits, equities and yields.
Economic stimulus: Biden’s economic response to the fallout from the pandemic comes in the form of a $1.9tn rescue plan in which economic stimulus will be distributed between a series of ‘initiatives’, including funding for the continued response to the pandemic, direct payments to Americans, government aid, an extension of jobless benefits, and a combination of other policy changes. Whilst the increase in stimulus is widely hailed as a positive post-pandemic move for economic recovery, the flipside is the potential subsequent rise in inflation as a result of increased employment, spend and demand, with direct impact to the stock market. The increase in consumer prices and decrease in the purchasing power of cash can have a direct impact on the stock market – causing stocks to fall, as interest rates are potentially hiked. In such a scenario, funds and asset managers will inevitably have to focus on shifting the balance from investment in stocks of heavily borrowed companies to those less impacted by the rise in interest rate.
Increase in minimum wage: Amongst one of the other policy changes that Biden plans as part of the economic stimulus plan, is to increase the minimum wage in the US from $7.25 to $15 per hour. The debate is over whether this will cause unemployment as businesses struggle to pay their employees, or more positively, increase consumption. Although the minimum wage would be brought up steadily and incrementally over the next 4 years, there are a number of potential impacts to purchasing power and buying behaviour, with onward impact to different market sectors affecting capital markets. For example, the food and drink, and hospitality industry – the rising costs to food outlets of paying their workers more, would need to be offset by an increase in revenue through increased consumer goods and menu prices. The question is whether consumers will be able to afford the higher prices without reducing demand, and the direct impact this might have on already-struggling outlets. Rather than asking by how much the minimum wage will/should increase by, the more important question might be when. Waiting until well on the road to recovery from the pandemic will be key – to a time when consumers feel safe and eager to go back out into the world and spend again, especially after a period of saving.
The way in which alt data is being sourced and consumed is changing after the pandemic. Why do you think this is and what do you see happening further within the alt data space?
The alternative data market was gaining momentum and growing exponentially even before Covid-19, but the pandemic has turbo-charged this growth and many providers are benefiting from this trend by some margin. The audience has broadened with an increase in data-curious clients. However, many still lack the depth in understanding and capability to adopt the current suite of data products available from vendors into their strategies. As well as continuing to cater to those able to consume large amounts of granular data (such as quantitative investors), it will be key for vendors to productise for the incoming client base of recent alternative data adopters, and help further democratise the alternative data space. Making data more accessible is one way, for example where S3 and SFTP may not be within the current lexicon of many, Excel and Tableau can be accessible alternatives. In addition, government bodies are starting to really understand and see the value in the real-time nature of alternative data. Data vendors must embrace this in order to turn “alt data” into just “data”.
What is your advice to funds hoping to get new systematic strategies into production quickly and more often?
There is a whole ecosystem of SAAS providers who are designing products that can process multiple data sets and allow users to rapidly set up strategies and explore techniques. This is not to say that a fund needs use these providers in a production environment, but having the ability to quickly test and tweak should not be overlooked even by the most data-sophisticated.
A portion of the industry still feel that advanced ML techniques such as Reinforcement Learning and Deep Learning cannot be applied to financial data effectively – do you agree? What are the main challenges in preventing this from happening?
It can be said that, up until now, advanced machine learning (ML) hasn’t had the impact that it has in other industries, such a healthcare. However that’s not to say that it doesn’t have the potential to be equally as effective when applied to financial data. The effectiveness of applying advanced ML techniques depends on the type of finance ‘problem’ or use case being tackled. There are lots of examples of neuro-linguistic programming (NLP) and deep learning being used in sentiment analysis and hedging. However, when we usually talk about the limitations of ML in finance, we’re referring to predictability of price/value of an asset. This is one of the biggest challenges to applying ML techniques because stock price encodes a vast number of different opinions from different participants, which can be driven by an infinite number of factors.
The main challenges we identify that prevent the effective application of ML to financial data are:
- Feature/factor amount vs amount of sample data. Machine learning is most effective when many factors or questions are programmed into the process for understanding and defining the output (e.g. returns of an asset). However, if there isn’t enough sample data to build an understanding from, then the accuracy decreases. One quick solution to this is to opt for a higher frequency e.g. move from quarterly numbers to daily. However, this could introduce a lot of ‘noise’ to the data. Many individuals are creating a large model that trains off many assets rather than just one to combine learnings from multiple assets.
- Capturing non-quantifiable factors. How could anyone have known that GameStop would increase by 400%+? You would have needed to visit Reddit and notice the momentum building on that stock. Even then, how can you capture this as a feature/factor for a model to build an understanding from? This is where advancements in NLP have helped but often this isn’t enough for investors to base decisions on alone, especially given that in finance, in particular, we’re better at capturing sentiment than an algorithm.
- Participants use of more complex factors and data driven-models. Many participants will use a simple linear regression and take the given prediction alongside any opinion they have about the value of an asset to make a final decision. However, if the entire process was driven entirely by a more complex algorithm, we’d see the increase in effectiveness of machine learning as a whole. There are hints that we may see this more in the future, with companies such as numerai that allows its users to use more complex data (aside from market data such as PE values) and make predictions on a stock price. (In this scenario, human opinion is still incorporated in the process but shifts from being applied at the end of the process to inform final decisions, to being applied just at the start to determine which factors to observe in the first place. If these are well-selected then we can rely on the decision made by the algorithms without the need for human opinion tooo.
You can hear directly from Suraj as he joins us as a speaker at AI & Data Science in Trading Online on March 16, 10:45 AM Panel – Shifting attitudes to alternative data.