Interview: Stuart Kozola, Global Head of Finance, Mathworks
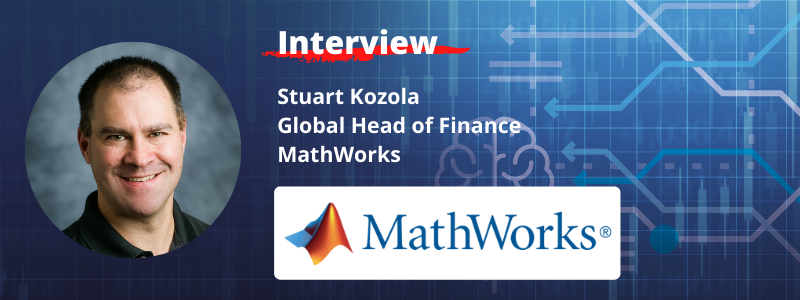
What do you think are the biggest challenges facing data scientists/AI experts/quantitative investors in 2021 post Covid-19 and why?
Post Covid-19 poses both challenges and opportunities for quantitative investors. On the challenges side of things, using historical data to develop new investment strategies will be challenging. The data set spanning 2020 and for the foreseeable future will not look like the data of the past as there are clear differences by industry sector (i.e., technology vs airlines) for example and new investors in the market (i.e., reddit wall street bets and their influence on Game Stop). Investors that are not seeking out new sources of data, such as alternative data, or build in risk management for events like game stop will have a harder time generating sustainable alpha without substantial downside risk. Markets will correct over time, the economy will rebalance to a new normal, and those who get out ahead of it will succeed. Success will come not from just applying AI/Machine Learning or traditional statistical models, but from a deeper understanding of causality and the dynamic behaviour of market participants. Investors looking to generate alpha will find success in diversifying their data sources for generating strategies, building their models on more than just price and fundamental data, but considering the dynamics of the market participants that lead to order flow. I think we will see more advances its alternative data set use, dynamic system models being deployed to explain order flow and market participants behaviour. But success will also come from being able to rapidly build new investment models and deploy them to production use. Those that embrace this trend adapt, managing risk, and succeed.
Don't miss new reports! Sign up for The AI Data Science in Trading Newsletter
How do you see Biden’s presidency and policies on key issues affecting capital markets in 2021? What should fund and asset managers be focusing on?
Biden’s agenda will clearly favour climate, sustainable energy, open science, fintech, and consumer protection and regulatory expansion based upon his views and current appointments he has made to key positions. Fund managers will need to identify and adapt their portfolios to align with these shifts and Biden’s policies and actions are declared. Opportunities with ESG, sustainable/responsible investing already are seeing increased investment during Trump’s administration, and this will get a boost under Biden. Climate will be a big deal with his administration, renewable energy will see a resurgence, and traditional energy will be challenged with new regulations or closing of access to resources under federal control. If you don’t have an ESG strategy today you should.
The way in which alt data is being sourced and consumed is changing after the pandemic. Why do you think this is and what do you see happening further within the alt data space?
Alternative data, from cell phone traffic records, to satellite images, to new market sentiment sources are becoming more mainstream and considered less alternative than a few years ago. Traditional finance data vendors are partnering or expanding their offering to new data sources and offering their platforms for other to sell their data through them. We are seeing a change towards “platformization” of data, were there will be a few dominant platforms that provide a marketplace for all sorts of data that brings both data owners and consumers together. This will provide more opportunities for companies to make money from their data at a larger scale and reach a broader audience, not just for investing but for other applications as well. There will likely be a push for more open and free data sets, particularly in the climate space under the new Biden administration and his commitment to science, and open science.
Cloud computing has been widely adopted in most sectors except financial services. Is this now changing, and if so, how will funds decide how and where to include external providers?
Every financial institution I’ve met with over the past 5 years has had a cloud initiative. Many of them were using the cloud for research, to develop, implement, and test out new product ideas before investing bringing the technology in house for use on sensitive/proprietary data sets. What we are seeing now is a broader acceptance by regulators and the cloud vendors on the level of security and protection the cloud offers are often better than internal systems. And the focus on cloud vendors on adopting multicast technology will further advance the viability of cloud for production use. As with any decision, elements that will move to the cloud will be those that are cost effective to do or those they provide capability not currently available in house. Data management and processing application will likely become more cloud driven as the scale and variety of the cloud here is already demonstrated. Machine learning models are requiring more computational resources and this is an area where cloud will be more advantageous to use as well.
ESG and sustainable investing is a topic that is becoming increasingly relevant in the current climate – do you believe ESG data can be used for as an alpha generation tool rather than just a risk management process? What changes are you seeing in how ESG data is being used?
ESG should be considered as a third dimension to be added to the traditional view of risk vs return. ESG preferences for investors will become more important a consideration to selection of assets to invest in. Inventors will decide portfolios based upon ESG-risk-return, portfolios that they view as aligning with their preferences. Considering ESG in this way allows it to be both a risk management tool, but also alpha generation. There are already mixed results in ESG studies for alpha out there, and there will continue to be as the area is new and use of ESG data is diverse. The challenge to overcome will be in using and defining the right ESG parameters as an additional filter for the investment universe selection, and the time impact they may have on the longer-term performance of the firm. ESG likely will have a longer delay before manifesting in alpha in a causal way, and ESG data is sparse and not well-defined today which leads to a lot of inconsistency. The trick for fund managers is to identify the ESG factors that influence performance and capture them before the broader market finds them. But that is already what they do in their quest for “alpha”. So, it’s not new, just another dimension to consider when building strategies.
A portion of the industry still feel that advanced ML techniques such as Reinforcement Learning, and Deep Learning cannot be applied to financial data effectively – do you agree? What are the main challenges in preventing this from happening?
Can you give some concrete examples where you’ve seen this work successfully? This is an understandable feeling. The challenge with financial data is that it reflects complex human systems and as such often sparse in causal information and includes nonstationary and regime shifts. This challenges modern ML to identify sustained patterns to use for predictive modelling that beat traditional ones. I agree that it can be challenging for Deep Learning and Reinforcement learning to be applied to traditional financial time series data for the reason just mention. This is particularly true when looking at price series which are really “incomplete” data sets. They don’t contain the causal factors leading to the order flow that drives the price dynamics observed. However, when considering a broader set of data, both are seeing successful use when you include non-structured data sets. Natural language processing use of DL/RL has seen success for extracting sentiment, entity identification and relationships, and ESG factors are often extracted from non-structured data. Using images to identify retail traffic volumes use these methods to create the signals used for other models. So, applications of DL/RL are there in many cases in extracting information used in more traditional models, such as portfolio optimization. DL/RL will continue to see more use in generating signals or factors from alternative and non-structured data sets and used as part of the data for investment decisions at a broader scale.
What is your advice to funds hoping to get new systematic strategies into production quickly and more often?
Getting new strategies into production quickly with higher velocity requires adoptions of new technologies and processes. There are new buzzwords around AIOps, ModelOps, and MLOps that talk about operationalizing models. Behind all of them are adoption of agile methodologies combined with continuous deployment that have come from traditional software development and data management (the DataOps and DevOps buzzwords go here). DataOps and DevOps teams have evolved with agile processes and new tooling to support the workflow. Establishing a similar one for modelling to production is where investments are occurring today. The balance that needs to be achieved is different in finance and data science as we need to democratize models to be able to be created from business domain experts and deployed with little (re)coding as needed. Taking a model created say in a spreadsheet and deploying that to production use is not the answer though as it doesn’t scale and hard to validate and manage. What is needed to achieve high velocity are tools that can be used by domain experts and generate code for use in production automatically, with supporting documentation and model lineage for risk management that also fit into modern DevOps solutions (since ultimately strategies end up as computer programs). Tools that generate production ready code and self-document from workflows performed in interactive tools that can run on CPUs or GPUs and scale to the cloud, often called no-code or low code solutions, offer this promise but few work seamlessly. But this is why I am at the MathWorks today, these are the tools we are developing and have so for over 30 years that enable mathematicians, scientists, engineers to take their ideas into production in a matter of hours not days. Bringing technology close to the domain expert in a form that solves business problems is a passion of mine. I enjoy the intersection of technology and finance, which is also why I enjoy the AIDST conference, it provides a good balance of both though leadership with applied technology.
Hear from Stuart, as he joins us AIDST Virtual, March 15 - 16, on the 1:50pm EST panel as he joins other industry leaders to discuss 'Utilizing advanced ML techniques – Reinforcement learning, NLP, Deep Learning'.