Interview: Rebecca Kelly, Technical Evangelist, KX
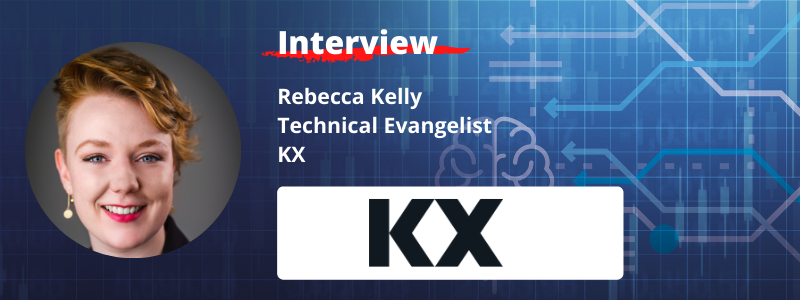
What do you think are the biggest challenges facing data scientists/AI experts/quantitative investors in 2021 post Covid-19 and why?
One of the biggest challenges facing Data Scientists in the post Covid-19 world will be the continuation of remote working and the communication difficulties posed by this. Getting stakeholder buy-in, communicating results and data democratization efforts will all rely heavily on data visualisation and presentation efforts by Data Scientists.
Don't miss new reports! Sign up for The AI Data Science in Trading Newsletter
Cloud computing has been widely adopted in most sectors except financial services. Is this now changing, and if so how will funds decide how and where to include external providers?
In my role as Technical Evangelist with KX I spend a lot of time talking with our clients, and understanding their needs and data strategies. Two years ago approximately 19% of our financial services customers were considering cloud adoption, and now in 2021 50% of them have implementations planned for the coming year so this is definitely changing. Most organizations within financial services are adopting a hybrid-cloud approach and quant research teams are often some of the first in-house adopters. This is partly for cost purposes as quant workloads can often be "spiky" and also because of the freedom these environments offer researchers in terms of tooling, scalablity and flexibliity.
What is your advice to funds hoping to get new systematic strategies into production quickly and more often?
Focus on optimizing your infrastructure and creating an end-to-end data pipeline that can be adaptive and responsive to new datasets. During our panel session on Monday we discussed this in depth, but fundamentally reducing that time-to-value of a new dataset is critical to capturing edge and driving continued success. In terms of accelerating the process of pushing strategies into production, I've seen a lot of organizations have success by employing agile cloud-based infrastructure. The benefit of the cloud infrastructure is you can run services concurrently while testing and failover rapidly in the event of errors.
Data engineering is oft a topic that can be ignored when dealing with AI implementation – what is important for an organisation to consider in regards to an infrastructural data strategy to support advanced analytical investment outcomes?
There are two key areas I would advise organizations to consider with respect to infrastructural data strategies and some questions that should be considered.
- Firstly, scalability is crucial because you have to be able to adapt to busy markets. It's also important to consider the scalability of your own operations too. Questions to consider are: How will this pipeline respond to increased load? Where is the limit of scalability with this approach? If we are amazingly successful, how would this system scale with our increased operations?
- Secondly, flexibillty is an absolute necessity to ensure your infrastructure is working for your team, and not the other way around. Does this approach allow our teams to use all the tools they know and love? How many ways can data be provided by this infrastructure (Stream/Batch/GUI/Quant Sandbox)? Will this system be able to adapt and change as our business changes? How straightforward is it to extend the infrastructure?
Finally, as guidance beyond the above considerations I would say that cloud-based systems are a quick win for scale and microservice based architectures offer flexibility for future changes and growth.
What is your biggest professional achievement to date?
I'm very proud of having received the 2020 Tech Evangelist of the year award from the Women in IT Awards organization. It was a great acknowledgment of my efforts since becoming a Technical Evangelist and a nice highlight in an otherwise quiet year!