Deciphering Chat GPT: a gate to augment financial intelligence
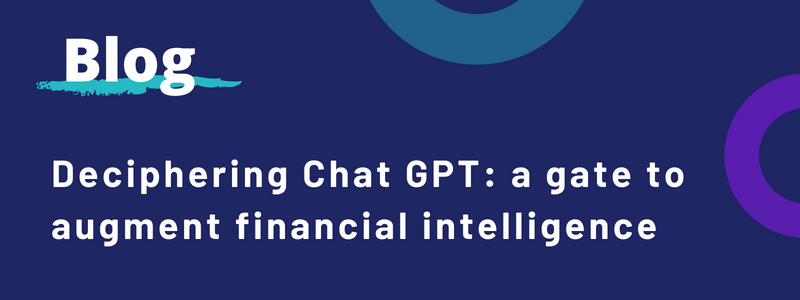
Behaviourally, cognitively, reversible, mutually exclusive are some of the properties of “a questions game”. In a word, confusing, unless we know the nature of the source of the answer. Having said that, speculations, and curiosity about the limits of Chat GPT, conducted the sceptical to running all sorts of tests to check how well it performs with logico-mathematical, sematic, and ethical requests forgetting that it is not what is achieved but how it is achieved that matters. This how are the queries, to me semantic well-worded questions, which -by definition- require understanding and experience from both the meaning and the context.
Assuming that the reader will be well acquainted with the nature of Chat GPT, I shall not describe it in detail here. Yet, it is worth stressing that, from its humble beginning, it has shaken the foundations of the ground-breaking works of its predecessors displaying superior common-sense reasoning and reading comprehension skills shaping intelligently “prompts,” where “selective attention” comes into play.
As witnessed, the scope of research in language models applied to investment purposes has grown exponentially beyond its traditional use, proclaiming Chat GPT a seminal development in its own right, and certainly a driving force that revolutionizes the financial industry.
In this post, I would like to contribute with a concise, and hopefully compelling illustration of how Chat GPT could be a gate to augment financial intelligence, and during our journey provide a glimpse of the transformative power and potential applications.
Being a sophisticated model empowering semantics, Chat GPT promises far more personalised and relevant content for all users. Impactful as it is, early adopters – accredited investors, traders, and market players- are accommodating to this transformative idea, pondering about ways to integrate cautiously Chat GPT into their workflows to do their jobs better, smarter, and why not “striking gold”. Still, in a business driven by value-add nobody wants to admit that Chat GPT could outperform their analytical skills and eventually – though unlikely- replace them.
Don't miss new reports! Sign up for Quant Strats Newsletter
Stigma aside, originally designed to augment human abilities articulating answers in many domains, Chat GPT applications are wide-ranging. As a way of democratising financial knowledge, it can be utilised to furnish investment advice and information to a broader range of individuals through a conversational mode, making investment information more accessible and comprehensible particularly to amateur investors and nonfinancial professionals who are lacking the resources and expertise to “make meets end”. In this respect, Chat GPT could mitigate information asymmetry in the investment process.
In banking, it can be employed to aid users “analyse” large amounts of data, allowing banks to become “cognizant” and make better-informed decisions, “examine” customer data and transactions to identify unusual activities, to name just a few. In the FinTech scenario, a primary application is performing textual analysis of financial articles, daily reports on the stock market, and social media posts or tweets to aid investors in generating strategies to predict a particular stock's future returns or an entire portfolio. Up to here and for a “good reader”, nothing too different for what prior models could do.
The “how” and the “what”
The enigma lies in starting with the quality of the semantic-worded question or to put it simple “query”. Intelligently selective wording, as mentioned is key to accurate responses. The more specific and centred questions are the more refined and “assertive” the response. The amount of information “less sometimes is more” also applies. Because behind the scenes, keeping things simple and with enough information, attention is placed on specific words which will provide a reasonable answer. “Positional information” turns to be relevant. Still, the “how” (for a more in-depth search) helps to predict with high confidence that what follows is a question, therefore answerable.
What about “nuances”? How might we learn to attend to? It is true that one only needs to write a prompt in plain language to obtain the issuing text. Apparently, the clue also lies in our ability to express the “subtleties” simply because in language “attention” matters.
The “ensemble approach”.
To have a thorough understanding of high-level written documents, such as annual reports, investors presentations, and earnings conference calls, is vital. Therefore, instead of devoting hours to analysing and interpreting large amounts of data to identify patterns, summarise ideas and key concepts, why not let us be assisted by Chat GPT?
Earnings conference calls (from now on ECCs) are what some might call “a source of intel”, if you know what you are looking for. Due to the richness of the content, one must be aware of, first and foremost, the nature of the query (and this applies to all cases). As previously stated, human beings tend be divergent when posing questions, so for Chat GPT, the more specific or detailed the question is, the more “accurate” the response will be. Although Chat GPT can formulate coherent and full responses, it may not be able to understand the nuances of a question. So, one must be extra careful when using it for professional consultations.
“Dissecting” a piece of discourse -an ECC- allows a more comprehensive overview of, for instance, the management team’s remarks and Q&A session. So, it will be sensible to apply the same logic when focusing on the crucial aspects discussed by the management. Perhaps here, listing is the best option rather than summarising. Fine-tuning is an essential strategic approach. It’s unlikely that anyone will use anything that Chat GPT “utters” word-for-word. Instead, the best way is to issue descriptive prompts, refine them and adjust the responses you get back.
In our example (and extending it to other cases), can we say that ECCs are enough? Surely not.
Hybridity seems the solution. We can go further into our analysis and include more data in our corpora. Blending strategies including SWOT, risk analysis, historical data analysis, create a portfolio of stocks, “interpreting” news articles about the company and analyst ratings seems suitable for Chat GPT which should be seamlessly integrated with coding to evaluate the performance of stocks and data in “real-time”. It is true that you may use Chat GPT to conduct a complete financial market analysis, hence being skilful in the “art of talking” to an AI and understanding the method you ask is a must, if the goal is to “augment financial intelligence”.
A warning: not all data is in real-time since Chat GPT allows queries until 2021. Yet, it might work if provisions taken. What I mean is that if notable information transpired within the company or industry sector is missing it may result in a setback.
Another issue that raises more and more concerned is trying to elucidate if a company meets ESG standards. Assessing a firm’s credentials might be a perfect task for Chat GPT. Again, “sauce for the goose…”. A swift reminder: Chat GPT refuses to answer questions out of its knowledge.
“Reading between the lines” the moat of a company, paves the way to stay ahead of competitors, and eventually gathering and crosslinking all the data, leads you tiptoeing into “generating alpha”. How about technical analysis indicators? “Buying the dip” sounds tempting. But that is a whole new chapter.
Once natural obstacles are overcome, though not “perfect” yet it is expected that Chat GPT to level the playing field in financial engineering landscape.