Data ethics in AI hedge fund trading
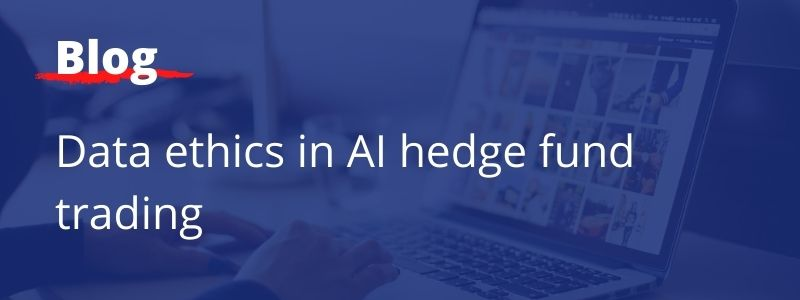
As powerful as AI is, it doesn’t have a data conscience.
Hedge funds have long been using computer models to make the majority of their trades.
AI now offers asset and investment managers autonomous algorithms and systems, reducing use of data scientists to help with manual and moderating input.
The ability to analyse large amounts of data at super-fast real-time speeds is a genuine gift.
Don't miss new reports! Sign up for AI & Data Science Trading Newsletter
AI can deliver insights from a host of information sources such as alternative data sets compiled from wide-ranging ‘unofficial’ sources.
These could be credit and debit transactions, news articles, images and social media posts, public records, web traffic, web searches, biometric data, mobile device tracking, satellite imagery, geo-spatial information, supply chain and logistics data, and more.
All this is very different from regulatory filings, press releases, and management commentary produced directly by a company itself.
And thanks to machine learning, AI is feeding back increasingly accurate predictions.
With raw computing power growing daily, AI can offer ‘plug and play’ actionable trading signals in hours instead of weeks, be it from satellite imagery, the internet of things, global capital flows, point of sale systems, and social media.
AI includes the power of natural language processing (NLP) too. This can parse earnings call transcripts to reveal sentiment around important fundamental key drivers, including Margin Forecasts, Market Position, CapEx, Capital Returns, Guidance, Wages, and others.
During the pandemic, Blackrock, for example, employed NLP on research documents to glean insights from analysts many of whom were relatively slow to update their earnings estimates for the first quarter of 2020.
Use at will?
- Rich pickings – and the systems rarely come with a "no responsibility" or “use at your own risk” disclaimer. But many risks that come from the use of AI do require the input of ethical values and principles. Because how do you:
- Control the lack of auditability of AI?
- Measure data quality?
- Monitor AI systems’ discriminatory decisions?
- Use AI in unexpected events with no historical data available?.
- Ensure adherence to current protocols on data security, conduct, and cybersecurity on new untested AI technologies?
- Make allowance for social purpose so as to not judge unfairly?
- Avoid bias – be it human or AI systems own biases, derived from flawed training datasets, processes and models?
- Define responsibilities of the third party provider and the asset management firm using the service or tool?
Regulatory implications grow
These gaps are not going unnoticed by regulators. Regulators have woken up because the use and possible informational advantage of such alternative data sources and dark analytics raises difficult questions regarding propriety, privacy, fairness, and ethics. It’s why we are now seeing increased engagement from regulators with respect to AI, particularly in the financial services arena. But it’s a complex and evolving area, and it’s changing so fast it’s difficult for regulatory authorities to keep pace.
Currently there is limited specific AI legislation applicable to financial institutions, outside the EU’s General Data Protection Regulation (GDPR) and MiFID’s conflict of interest guidelines. Similarly, the EU Ethics Guidelines only ‘recommend’ that the trustworthy use of AI should be “lawful, ethical and robust”.
Now, however, the FCA has publicly stated that the rise of Big Data has “raised significant questions about the adequacy of the traditional liberal global frameworks for competition and regulation”.
They are aware that if customers do not understand how companies use their data, the data economy as a whole will be harmed and they have queried whether the traditional approach of financial services regulation is too liberal in this context.
No AI system has consciousness or even a modicum of the flexible intelligence humans use to solve the issues we grapple with on a daily basis. Such as what constitutes insider trading? If an investment bank, for example, uses vendor data with proprietary method of combining the data into a valuable new source, creating information asymmetry and unfair advantage, how can it be regulated?
These are thorny issues – and as yet, unresolved.