AI, Quant Tools & Portfolio Optimization: A Smarter Approach to Investment Strategies with SimCorp
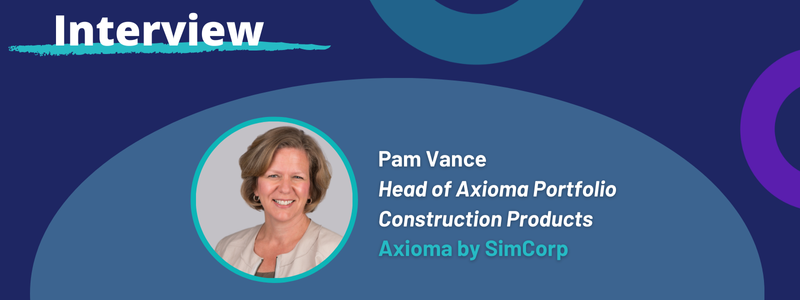
Pam is set to speak at Quant Strats 2025 on the 13:30 PM panel: Leveraging Best-in-Class Tools for Portfolio Allocation and Optimization. The panel will take place on March 11 at The Quorum by Convene, New York. Register here to join Pam!
Please give us a little introduction to your current role and what you do
I lead the team that develops our portfolio construction solutions at SimCorp, a front to back investment management platform. These products, which are used by portfolio managers across asset management, wealth management, hedge funds and investment banks, are under the Axioma brand.
Everyone is talking about AI, but what are some of the practical applications you see in portfolio construction?
We recently ran a webinar on this very topic. In it, we talked about two ways that the Axioma team is integrating AI into quant solutions:
First, in wealth management, the Axioma Portfolio Optimizer employs machine learning to help managers handle thousands of portfolios efficiently. It provides a WealthLens analytic score that identifies which portfolios need attention and learns from manager behaviour to enable faster, more accurate decisions without manual review.
Second, the Optimization Co-pilot serves as an AI interface between portfolio managers and the Optimizer. Instead of building complex optimization strategies manually, managers can express their objectives in natural language (like "reduce tracking error to 1.5% while lowering large-cap overweight to below 100 basis points"). These are translated into optimization API calls while maintaining transparency by displaying all constraints and selections used. This streamlines the implementation process, allowing managers to focus on critical decision-making rather than the mechanics of the problem set up.
So, you’re making optimizers more accessible?
Yes, even more so than they are now. It’s one of the big misconceptions about optimizers. We hear a lot that optimizers are too complicated and that one needs a PhD to use an optimizer. It’s true that you probably need a PhD to build an optimizer, but most optimization problems are relatively simple to articulate and implement. For example, ‘I want to maximize expected return as defined by my alpha forecasts after accounting for transaction costs, while not exceeding a tracking error of 3%, having no more than 5% active weight in any sector or individual stock, and no more than 5% turnover.’
What are some other misconceptions about optimizers?
There are many! In fact, we wrote an article on this. The main ones are that optimization is only for equities (when in reality it can handle any asset class), that using an optimizer isn’t going to improve performance (we’ve proven that it can – and how it can) and that optimization is scary because it’s such a black box (it’s not). Finally, fundamental managers – they think quant tools are not at all applicable to their strategies, which cannot be further from the truth.
That last point, we hear this too. At Quant Strats, we always discuss the challenges and opportunities of blending quant and fundamental strategies and this is always a popular topic – what are some of the advantages?
There is a lot of benefit for fundamental managers to use quant tools. For example, factor risk models provide a disciplined "second opinion" to help managers avoid emotional attachments to stocks and maintain objective decision-making. These tools are particularly powerful for understanding portfolio risk, especially revealing non-obvious risks and unintended exposures.
Quant tools also help managers speak a consistent language through ex-ante risk analysis and ex-post factor performance attribution. This alignment helps demonstrate value and justify management fees.
Portfolio optimization tools – as discussed previously - can help managers make more informed portfolio construction decisions. Finally, quantitative tools can enhance performance by identifying unintended bets and negative payoffs through risk analysis and return attribution. This allows managers to maintain their conviction on individual investments while avoiding inadvertent drags on performance.
You’ll be speaking about optimization at the Quant Strats event on March 11, but tell me more about the role of a risk model which readers may be less familiar with.
Effective portfolio optimization depends on two key components: a robust optimizer and a comprehensive risk model. Risk models are crucial because they measure how each stock is exposed to multiple risk factors. These factors include industry, geography, currency, and investment style characteristics.
The risk model captures both the individual volatility of these factors and their correlations with each other. This in turn determines the portfolio's expected volatility and active risk. Understanding these risk dynamics serves two essential purposes: it enables the construction of efficient portfolios and helps predict which factors will influence the portfolio's performance relative to its benchmark.
Download the full program here.
Finally, what are the ways the Axioma optimizers differ from their peers?
Portfolio optimizers are either general-purpose (powerful but complex to use) or special-purpose (easier to use but with a narrower set of capabilities). The Axioma Portfolio Optimizer combines the strengths of both, featuring a sophisticated engine that can handle mathematically complex construction scenarios with an interface that speaks the business language of portfolios.