Unlocking the Full Potential of Machine Learning: Insights from Industry Experts
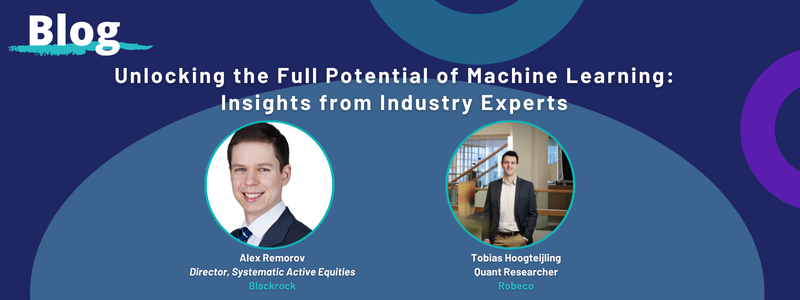
Machine Learning (ML) continues to revolutionise various industries, driving innovation and efficiency. Unlocking the full potential of machine learning hinges on the wisdom of industry experts who bridge theory and application. Featuring insights from two esteemed industry leaders: Alex Remorov, Director, Systematic Active Equities, Blackrock, and Tobias Hoogteijling, Quant Researcher, Robeco. Their expert analysis and forward-thinking perspectives provide invaluable depth to how ML is revolutionising the world. Read on to discover their unique viewpoints and strategic recommendations.
Promising Industry and Impactful Applications
Finance: The finance sector has seen significant advancements through ML, particularly in fraud detection and risk management. Algorithms can detect unusual patterns in transaction data, flagging potential fraudulent activity in real time. Moreover, ML models are used for algorithmic trading, where they analyse market trends and execute trades at optimal times, thus maximising profits.
Quality Data: The foundation of any successful ML project is high-quality data. Organisations must ensure their data is accurate, relevant, and comprehensive. This often involves cleaning and preprocessing data to eliminate errors and biases that could skew results.
“The advantage of a data-driven approach that it is, by itself, able to identify which approaches work well in which specific area. For example, machine learning techniques are able to determine for which assets certain variables play the biggest role. However, we believe that this requires a carefully designed and prudent approach. It is important to make sure patterns detected by ML algorithms are sensible and have external validity.”
Tobias Hoogteijling, Quant Researcher, Robeco.
Skilled Workforce: Having a team with the right skill set is crucial. Data scientists, ML engineers, and domain experts must collaborate to design and implement effective ML solutions. Investing in training and development can help bridge any skill gaps within the organisation.
Clear Objectives: Defining clear objectives and KPIs from the outset ensures that ML projects stay aligned with business goals. This clarity helps in measuring success and making necessary adjustments throughout the project lifecycle.
Key Factors for Successful Implementation
Scalability: Successful ML implementation requires infrastructure that can scale. Cloud-based platforms, such as Google Cloud ML Engine and AWS SageMaker, provide the necessary resources to handle large datasets and complex computations, making it easier to deploy and manage ML models.
“Key aspects include the technology stack, data quality and quantity, and relevant expertise. A scalable, flexible technology stack enables rapid experimentation and analysis, efficiently processing data and presenting clear results. Machine learning, especially deep learning, requires large, high-quality datasets. Additionally, expertise in both technology and industry-specific knowledge is crucial for effective analysis and faster adoption of machine learning.”
Alex Remorov, Director, Systematic Active Equities, BlackRock.
Overcoming Challenges
Data Privacy and Security: One of the primary challenges is ensuring data privacy and security. Organisations must comply with regulations like GDPR and implement robust security measures to protect sensitive information. Anonymising data and using secure data storage solutions are effective strategies.
Integration with Existing Systems: Integrating ML solutions with legacy systems can be challenging. A phased approach, where ML is initially used to enhance specific processes, can facilitate smoother integration. APIs and middleware solutions can also help bridge the gap between new and old systems.
“Key aspects include the technology stack, data quality and quantity, and relevant expertise. A scalable, flexible technology stack enables rapid experimentation and analysis, efficiently processing data and presenting clear results. Machine learning, especially deep learning, requires large, high-quality datasets. Additionally, expertise in both technology and industry-specific knowledge is crucial for effective analysis and faster adoption of machine learning.”
Alex Remorov, Director, Systematic Active Equities, BlackRock.
Cost and Resource Constraints: ML projects can be resource-intensive, requiring significant investment in technology and talent. Organisations can address this by starting with small, pilot projects that demonstrate value before scaling up. Additionally, leveraging open-source ML frameworks and tools can reduce costs.
Conclusion
The potential of machine learning is vast and varied, with significant impacts already realized in industries such as healthcare, finance, retail, and manufacturing. By focusing on quality data, skilled teams, clear objectives, and scalable infrastructure, organizations can successfully implement ML. Addressing challenges related to data privacy, system integration, costs, and change management will further unlock the full potential of this transformative technology. As ML continues to evolve, its ability to drive innovation and efficiency across sectors will only grow, heralding a new era of technological advancement.
“It usually takes some time to build the right system for machine learning. Such a system usually requires an investment, both in terms of technology spend, and in terms of people spend. To get more comfort before fulling committing it can help to do smaller scale experiments/tests to understand how machine learning would be beneficial in a certain area. There is also an element that the first model produced by the machine learning system is often not particularly insightful. As such, iterating on the model and being able to enhance it becomes important to fully realize its machine learning potential.”
Alex Remorov, Director, Systematic Active Equities, BlackRock.
October 8th at Quant Strats, industry experts Alex Remorov, Director, Systematic Active Equities, BlackRock, and Tobias Hoogteijling, Quant Researcher, Robeco, will provide invaluable in unlocking the full potential of ML. As vital pillars of stability and opportunity in finance, these markets are constantly evolving, driven by technological advancements, regulatory shifts, and changing investor preferences.
PANEL: 2.00 pm PANEL: Where is the potential of Machine Learning best realised?, during the discussion, we will delve into the practical applications of ML, probing whether its limits lie in internal enhancements of daily tasks or if it's breaking ground in generating Alpha. Join us as we unravel the complexities and opportunities in the ever-evolving landscape of Machine Learning.
Don't miss this opportunity to gain valuable insights and stay ahead of the curve. Download the agenda and sign up now to secure your spot!