Victor Naroditskiy: Gen AI will be spreading into areas where it delivers concrete and measurable business value
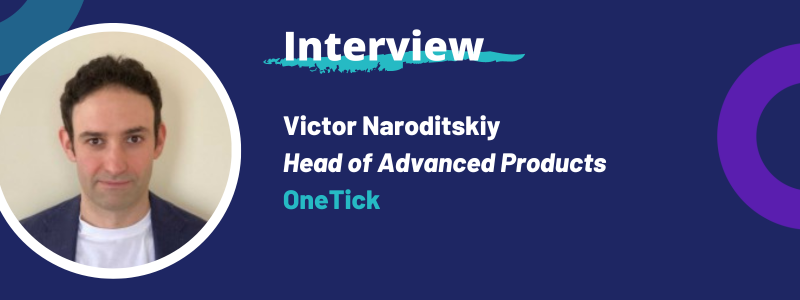
Please give us a little introduction on your current role and what you do
I am managing ML and tick data analytics products that enable quants to take their research to the next level in terms of the speed of trying and validating ideas that involve tick-by-tick market data.
What do you consider your biggest professional achievement to date?
Building a team that consistently and successfully carries out some of the largest and most complex implementations involving analysing hundreds of billions of ticks per day for regulatory purposes and quant research.
Don't miss new reports! Sign up for Quant Strats Newsletter
What has been your /your firm’s top 3 priorities for the coming year?
We want to grow the ML and quant analytics business in the following three areas:
• Making quant research easy with a highly-performing pandas-like API
• ML solutions such as pre-trade TCA, surveillance alert scoring, abnormal behavior detection, and volume/volatility forecasting
• An end-to-end MLOps platform and an ML lib for machine learning with market data
What do you think are the biggest challenges facing data scientists/AI experts/quantitative practitioners for 2023 and beyond?
Availability of high-quality harmonised data and the technology to efficiently analyse high volumes of data is one of the challenges. The challenge is even more pronounced when it comes to real-time data. Related to this: the ability to reproduce results published by others is highly limited.
Market and political uncertainty over the last year has seen unpredictable outcomes for some quant firms – how do you think quant firms can prepare for increased uncertainty to come and manage the 40-year inflation high that was seen in 2022?
Optimising with respect to different scenarios some of which include extreme inflation rates is a must.
What is your advice to funds hoping to get new systematic strategies into production quickly and more often?
Have a robust framework in place for backtesting and algo monitoring. Use a vendor that provides the relevant market data and backtesting framework as managing in-house may be expensive both in terms of costs and maintenance.
ChatGPT is everywhere and being used everywhere. How do you see quant funds using this new technology and what advice can you give people using it?
There is not a clear application of ChatGPT to trading. Similar to large language models that are trained on enormous amounts of data, time-series based models trained on say 10 years of tick-by-tick level 1 and 2 data may appear that will be able to reason about time series (e.g., make predictions on traded volume, volatility, price moves).
a. What are your predictions for generative AI in the coming years?
Gen AI will be spreading into areas where it delivers concrete and measurable business value automating many of the language and operations related activities.
NLP continues to be a big area of interest during our research – is the industry really using it to its full potential? Where else can we go with NLP and have you seen examples in other industries that we can learn from?
Efficient data retrieval and analytics using natural language rather than code are two areas where NLP can deliver enormous value.
Quant Strats London
Catch Victor Naroditskiy at Quant Strats on the 24th of October at The Park Plaza Victoria, London. He will presenting on "Quant research and ML with tick data" at 2 pm on our AI/ML Innovation track. Victor will be exploring how easy it is to implement typical market data analysis use cases that arise in quant research, applying machine learning to tick data with a purpose-built library and infrastructure and so much more!
To learn about Victor's presentation and the full program
Don't miss your chance to hear from 50+ speakers at Quant Strats on the 24th of October, register here today!