Leveraging Quant and Systematic Methods in Fixed Income Investments
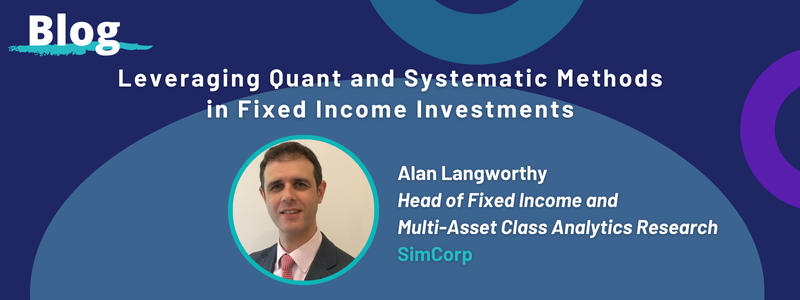
In the realm of Fixed Income Investments, the adoption of quant and systematic methods is revolutionising portfolio construction, risk management, and performance attribution. Alan Langworthy, Head of Fixed Income and Multi-Asset Class Analytics Research, SimCorp, dives into how these techniques can be effectively harnessed, the value of high-quality data, and best practices for funds aiming to swiftly implement new systematic strategies.
Alan’s responsibility is R&D for the Axioma portfolio analytics suite and his primary areas of focus are enhancing the Axioma Credit Spread Factor Model, performance attribution and liquidity capabilities on the integrated SimCorp One platform, which is designed to address the front-to-back needs of investment managers.
You mention your focus is on the fixed income space. Can you talk us through how quant and systematic methods can be used here?
Systematic methods in fixed income have a real benefit both pre- and post-trade. Portfolios constructed using a factor model – regardless of whether the portfolio is equities or multi-asset – can be optimised for minimal ex ante risk, which in many cases is a more direct way of achieving what is usually the desired outcome of a stratified sampling approach.
The advantage of the former approach over the latter is that it often results in lower tracking error, as it takes correlations between multiple risk drivers into account. Ex post risk and performance attribution can be presented in a way that more closely aligns with the investment decisions taken, leading to a better understanding of how a strategy is performing.
With so much data in the market right now, where do you think the most value can be found?
As you rightly point out, value is not about the quantity of data, but the quality.
Until recently – especially at it pertains to the fixed income space – techniques for extracting high quality information from large amounts of lower-quality data were limited by technological capabilities. And while it’s certainly true that advances in technology like AI have enabled us to push the boundaries to extract more high quality information – like in the credit curve-building we did for the Axioma Credit Spread Risk Models – advancements can only be made when companies understand the balance of compute power and cost. Further, these companies need to be willing to prioritise investment in a more efficient and future-proofed tech stack.
Our clients’ operations and regulatory environment have become gradually more complex, and we need to help them shift from information and analysis to decisions and outcomes. Alan Langworthy, Head of Fixed Income and Multi-Asset Class Analytics Research, SimCorp.
What is your advice to funds hoping to get new systematic strategies into production quickly and more often?
The basic investment tenet of understanding what you are investing in still holds. Invest in factors, but make sure you understand how they are constructed. To do this, you need to really dig into the methodology and find out not only what the risk model can do, but importantly, what it cannot do.
Some of the questions investors should be asking themselves may include:
- Does your risk model align with the investment process/portfolio?
- Are you trying to make returns from something not explicitly modelled?
- If you’re leveraging specific risk, does your factor model handle this component adequately?
At Quant Strats, we always discuss the challenges and opportunities of blending quant and fundamental strategies, and this is always a popular topic. What do you think are the most important questions for quants when considering this strategy?
The data challenges in fixed income have made it very difficult for quants to develop similar systematic strategies as the ones we are used to from the equity world.
For example, we recently ran an analysis on three different ETF ‘systematic’ strategies. We found there are “smart beta” high yield funds that state that they are systematically identifying bonds with high quality or value by looking at balance sheet ratios from individual issuers. The question that quants, as well as the buyers of these products, should ask themselves is: Is this really a quantitative strategy, or is it just using fundamental data to identify securities with superior return potential?