Interview: Hamza Bahaji, Head of Financial Engineering and Investment Solutions, Amundi Asset Management
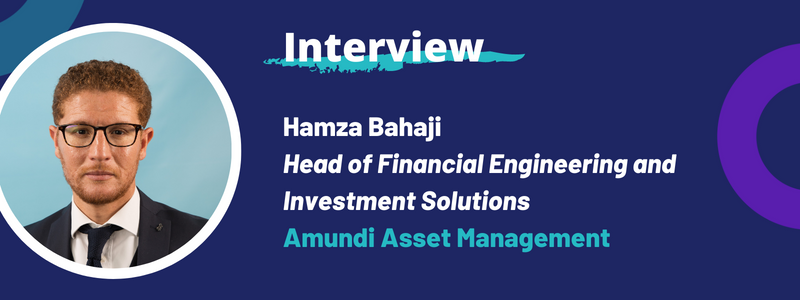
Prior to joining Amundi, Hamza spent 12 years with Natixis Asset Management. His latest role was Head of Engineering and Quantitative Research at Seeyond, the active quantitative portfolio management arm of Natixis Investment Managers. He started his career in 2004 at AON Hewitt Associates as Financial Engineer in the Investment Consulting department. Hamza holds a Ph.D in management science (Quantitative Finance) and a Research Master Degree in Finance from the University of Paris Dauphine, and a Master Degree in Applied Statistics and Actuarial Science from CNAM-ENSAE.
Don't miss new reports! Sign up for Quant Strats Newsletter
Aminah Hanif (Content Lead, Quant Strats): Hi Hamza, thank you for joining us today for this interview we are interested to learn more about you and what you do at Amundi Asset Management.
Hamza: Thank you so much for having me today. I am leading the financial engineering and investment solutions team at the systematic and passive investment division of Amundi. The core operating area of this team is the design of dedicated systematic investment strategies wrapped in ETFs or Mutual Funds. The subsidiary areas touch on financial engineering issue solving, including for instance the development of portfolio construction algorithms.
Aminah: What do you consider your biggest professional achievement to date?
Hamza: I believe that my primary achievement is the setup then the development of 3 quant investment teams during my carrier where I had the chance to collaborate with wonderful and bright quant engineers and PMs.
Aminah: What has been Amundi Asset Managements top 3 priorities for the coming year?
Hamza: The top strategic priorities of my firm are:
- Development of the Tech business unit which offers portfolio management tech solutions and trading services.
- Strengthen our leader position of ESG and Climate investment solutions provider in the market.
- Grow our market share in the European ETFs market.
Aminah: It's great to hear that ESG is at the centre of your priorities, and what do you think are the biggest challenges facing data scientists/AI experts/quantitative practitioners for 2022 and beyond?
Hamza: As far as I can tell, from an asset manager’s perspective, recent market events have proven very challenging to many sophisticated quant-driven strategies using AI/ML frameworks. The main underpinnings here were mainly endogenous consistency issues of the underlying models/frameworks (i.e. some latent factors overlooked and, therefore, failure to capture signals that would’ve helped predict those market events). That’s a big classic of quant investing. I believe the big challenge for quant experts focusing on the design of portfolio strategies is the development of sound model risk management processes with the aim of assessing a degree of confidence that one may put in the models’ predictions and, then, adjusting the construction of the investment portfolios accordingly. To address those issues one might find some inspiration in the exotic derivatives pricing discipline.
Aminah: What is your advice to funds hoping to get new systematic strategies into production quickly and more often?
Hamza: I think that quant funds should comply with this new paradigm change in the industry that significantly affects the whole value chain. From that perspective, it is crucial to embrace a client-centric (instead of product-centric) approach and build “cost-efficient” systematic solutions that primarily aim to address proven investment needs. This will help quickly break through AUM size hurdles and engage then in the virtuous circle where good investment outcomes attract higher inflows.
Aminah: ESG and sustainable investing is still a large topic, with many funds listening to investors’ demands and influencing their portfolio management. How do you see this progressing in the coming years?
Hamza: I emphatically believe that sustainable investing will gain momentum as most market participants are adding related objectives in their investment policies, with climate and environmental issues put at the forefront of their priorities. The main drivers of this transition will be the development of regulatory frameworks, particularly on the climate front, and higher awareness of investors of climate change and environmental risks. Some “purists” even believe that future market benchmarks will shift to the “green” stance, which means that, potentially, a risk premium for “brown” assets will metalize in the long term.
Aminah: Alternative data is still considered a source of alpha for many – what roadblocks do firms tend to come across in sourcing, cleaning, and using this data? How do you view the alt data market at present and where is it heading?
Hamza: Any source of information is still worth exploring for alpha generation as long as it is not made public extensively and used, on a very large scale, as an investment signal. Alt data does not escape the rule. It is unfortunate to see sometimes some NLP-based data advocated for alpha generation while the related information has been already embedded in asset prices. My guess is that the segment of alt data market dedicated to alpha generation is far from being mature and still has a very promising potential of development.
Aminah: A portion of the industry still feel that advanced ML techniques such as Reinforcement Learning and Deep Learning cannot be applied to financial data effectively – do you agree? What are the main challenges in preventing this from happening? Can you give some concrete examples where you’ve seen this work successfully?
Hamza: Saying that ML/DL technics are presumably ineffective when it comes to processing financial date is a strong statement from my point of view. I had the chance to apply some of those technics to address “real-life” investment cases and design investment solutions that are currently managed for institutional investors. One example that I can mention here is the design of alternative stock clusters that are used in portfolio construction instead of the traditional GICS clusters (which are widely used in the industry but have begun to present some heterogeneity issues). Unsupervised Learning and Data Clustering technics allow to build more reliable stock clusters for portfolio allocation in this regards.
Don't miss Hamza on the 13th October at Quant Strats Europe (Park Plaza Victoria, London) for his presentation on 'Alternative data - analysis and implementation of new data sources to maximise returns' and the unmissable Panel 'Training ML models fter Covid - reducing risk and increasing yield' with Andrea Nardon, Chief Investment Officer at Creed & Bear.
Download you free agenda here.
Subscribe to Our Free Newsletter
We respect your privacy, by clicking 'Submit' you will receive our e-newsletter, including information on Webinars, event discounts, online learning opportunities and agree to our User Agreement. You have the right to object. For further information on how we process and monitor your personal data, and information about your privacy and opt-out rights, click here.