Interview: George Patterson, Chief Investment Officer, PGIM Quantitative Solutions
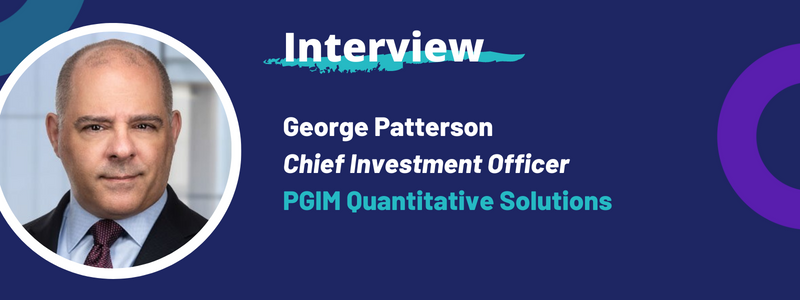
George Patterson is a Managing Director and the Chief Investment Officer for PGIM Quantitative Solutions. In this capacity, he oversees all portfolio management and research efforts for both the Quantitative and Multi-Asset Solutions teams. Join this unique opportunity where George closes the conference with a fireside chat on the challenges, opportunities and hurdles facing the quantitative investment community
Aminah Hanif (Content Lead, Quant Strats): Hi George, it's lovely to have a chance to catch up. Would you please give us a little introduction on your current role and what you do.
George: I’m the Chief Investment Officer for PGIM Quantitative Solutions, the quantitative and multi-asset specialist of PGIM, with $92bn in assets under management as of 6/30/22 and a 45-year track record of building systematic investment solutions. In this capacity, I oversee all portfolio management and research efforts for both the Quantitative Equity and Multi-Asset teams.
Aminah: What do you consider your biggest professional achievement to date?
George: I’d have to say that I’m most proud of the people that I have had the opportunity to mentor over the past 27 years. I’ve always enjoyed developing talent, and it’s very rewarding to watch the career trajectories of the people I’ve worked closely with as they’ve moved into more senior roles and see the influence that I’ve had on them as they’ve grown professionally.
Aminah: What has been your /your firm’s top 3 priorities for the coming year?
George: Given the events of the last few years leading up to the tragic events in Ukraine, most of the investors we talk to are very focused on the twin threats of volatility and inflation. In these challenging times, we’re helping investors to find ways of diversifying their portfolios and diversifying returns. Our models are built to exploit asset and regional opportunities, combined to give differentiated returns based on client needs, or to exploit inefficiencies in specialized markets. In this uncertain environment, it’s critical to remain agile and diversify away from a traditional 60/40 portfolio. Another area in high demand because of the uncertain road ahead is a focused portfolio of ideas and asset classes that are designed to perform well during negative equity market environments, while maintaining an overall positive Sharpe ratio. Lastly, on the volatility front is inflation protection – utilizing our suite of commodity and other inflation-sensitive strategies to build inflation protection solutions.
On a more long-term basis we are seeing demand for customization and personalization. Across the spectrum of investors, customized solutions are increasingly in demand. Our teams provide customized analysis and solutions to the most sophisticated investors and address the needs and requirements for customized solutions for investors’ unique challenges.
Aminah: What do you think are the biggest challenges facing data scientists/AI experts/quantitative practitioners for 2022 and beyond?
At a very high level I’d say that the major challenges facing data scientists and quantitative practitioners are threefold: 1) Storage, 2) Computing, and 3) Data. While we have made notable progress unleashing vast amounts of storage and computing capabilities, addressing data needs is critical to being successful in this business. One of the main challenges associated with markets is that relationships are often not stable over long periods, leading to varied regimes that occur at different points in time. We know that large amounts of data are necessary in order to model the relationships required to build quantitative success. However, that data must span multiple regimes rather than coming from a particular sample where the dynamics differ from what we are seeing today. The simplest example is to look at inflation. While we’ve seen multiple periods of high inflation historically, the most recent instance of high inflation occurred from 1973-1981. I suspect that many of the alternative datasets being used today do not cover multiple inflationary periods.
One of the ways that we attempt to address these challenges is to ensure that we understand the mechanism behind a particular investment signal. We need more than the observation that something works, or something is correlated with future returns, because it’s entirely possible that whatever is driving a particular signal is dependent on a particular regime. Our approach anchors quantitative processes on either behavioural or economic fundamental and this helps ensure that our approach is more robust out of sample.
Another important aspect of quantitative research is a shift in philosophy. Instead of trying to tweak parameters to improve the signals in sample, we must do as much as possible to discern under what scenario the signal fails in order to understand just how robust it is.
Aminah: The devastating situation in Ukraine has also had a huge impact on quant trading – what has this been and what are your predictions? What other geopolitical shifts are happening and how are they affecting quant investing?
George: Geopolitical shifts always move markets, and this is probably one of the most challenging areas for quantitative investors because in many cases, there is no prior event we can evaluate and benchmark a strategy to. But when you have a conceptual model that demonstrates the mechanism of action behind your investment approach, you can evaluate whether this process will continue to succeed in the future, given what we’ve seen in the past. If we understand why a signal has been successful, we can then decide whether a changed environment is likely to make it less effective. This way, you have a framework for assessing the efficacy of your approach. It’s by no means perfect, but it’s the best you can do.
Aminah: To what extent do you see the use of blockchain/crypto integrating into capital markets? As crypto is becoming more mainstream, how have hedge funds responded and what could be the potential impact on capital markets?
George: While blockchain technology is certain to transform the financial landscape (which is discussed in-depth in a recent Megatrends report published by PGIM click here to view more), I don’t think that crypto currencies today are a viable component of a diversified institutional portfolio. Take Bitcoin: In addition to the regulatory risk investors face, what was supposed to be an inflation hedge has actually developed a correlation with risk assets. Given the amount of retail interest, and the lack of large institutional interest, it’s likely that there are extremely profitable opportunities that can be found from a quantitative approach in the crypto market. While we have not to date develop strategies in this space, I can understand the industry interest, particularly among hedge funds. Our clients are generally large institutions, typically with very long investment horizons and relatively low active risk budgets. Due to the large number of challenges that we’ve seen over the past few years, the first question you have to ask yourself is, do you want to be in this space? We have not yet seen demand from our client base yet.
Aminah: What is your advice to funds hoping to get new systematic strategies into production quickly and more often?
George: I think many people that jump into this industry do not fully appreciate the level of technology that is needed to manage a modern quantitative investment process. This goes beyond sourcing and cleaning data, to developing sophisticated algorithms in a robust manner and then fully vetting and testing these algorithms before deploying them into production. The benefit of being a quantitative researcher is that your insights, which are delivered in the form of a code or algorithm, directly impact the P&L of your strategy. The drawback of being a quantitative researcher is that your insights, which are delivered in the form of a code or algorithm, directly impact the P&L of your strategy. So, any bugs, flaws in logic, or sequencing errors that could cause your code to fail can result in a material financial loss.
Aminah: ESG and sustainable investing is still a large topic, with many funds listening to investors’ demands and influencing their portfolio management. How do you see this progressing in the coming years?
George: With ESG investing at a crossroads, reliance on ESG indexes is no longer sufficient to give investors the exposure to the ESG metrics that matter most. Over the last decade asset owners have shifted significant portions of their portfolios to passive investments and ESG investing must now be incorporated into these passive investments. I think customization via enhanced active indexing alternatives that delivers performance in line with a benchmark but also permits flexibility to incorporate varied ESG preferences in portfolios will be increasingly in demand by investors.
Delivering this level of customization requires that quants have access to better data in order to identify “good” ESG investments. This means going beyond biased company public disclosure (because companies still typically disclose on issues where they excel) and using timely, forward-looking data to make real-time investment decisions (rather than looking in the rear-view mirror with lagged data). We may also need to adopt different analytical techniques, like using NLP, to uncover new ESG insights from non-traditional information sources to deliver alpha in ESG.
Data screening, scalability, and a discerning approach to stock classification can help to identify differentiated opportunities that indexing or fundamental investing would likely miss. This broader scope of opportunities provides for portfolio construction across a truly global ESG universe. By employing a differentiated stakeholder framework not bound by strict definitions, quants establish a holistic approach to analysing companies’ management and operations, their interaction with stakeholders, and their exposure to risk. This approach can serve an important role in uncovering value in the ESG space while navigating a potential bubble in ESG investing.
Aminah: Privacy and regulation surrounding the responsibility and ownership of data is still an area being discussed. What measures are you predicting will be put in place to navigate any foreseeable data privacy challenges while searching for alpha, and how can funds learn to navigate these regulations and policies?
George: I think privacy is on the mind of many regulators and there’s no doubt that this will become a larger issue in the future. I don’t have a crystal ball, however, it’s fairly clear that when you get down to a very granular level of an individual’s unique data, there will be challenges to how it’s collected and used.
Aminah: Alternative data is still considered a source of alpha for many – what roadblocks do firms tend to come across in sourcing, cleaning, and using this data? How do you view the alt data market at present and where is it heading?
George: Alternative data is certainly a growth area of quantitative investing. I think many areas that were once considered alternative are now considered mainstream, so I’m not entirely sure what the definition of alternative is anymore. I think the biggest challenge to alternative data is that it can very quickly garner widespread attention from vendors, but upon further analysis, it’s not immediately clear how valuable the data is. In many ways there’s a misalignment of incentives between data providers and clients: data providers want to license as much data as possible to their clients, while clients only want data that can help them deliver superior performance. This reminds me of a quote that I believe was originally attributed to Jack Trainer, and it went something like, “transaction costs must be 10%, because I have a line of people outside my door that have a strategy that back-tests with 10% alpha.” But nobody can deliver that once the strategy goes live. I’m sure there will continue to be a healthy alternative data market but finding alternative data that truly adds value and is not correlated with something that you already have is and will always remain one of the main challenges of quantitative investing.
Our research this year showed a lot more firms and practitioners talking about NLP than usual – why do you think this is? Where are seeing the optimal utility for NLP and where does it have the potential to go?
Technological innovations have given asset managers access to massive amounts of numeric and textual data on a timely basis. Significant advances in artificial intelligence allow for processing vast amounts of data effectively and efficiently for more relevant and timely insights into asset fundamentals and prices.
NLP allows asset managers to quantify previously qualitative data by, for example, assessing analyst conference calls and central bank statements that can open a window into sentiment and yield additional insights. Similarly, NLP can be helpful in analysing news media, which means asset managers no longer have to rely on individual companies to disclose information. The use of big data is undoubtedly here for the long term but how it is being used is much more in transition, and the precision with which it is used even more so.
In many ways, we believe that we are in the early innings of a data revolution, but more data does not always result in more usable information. It’s also crucial to note that additional data sources are often highly correlated with existing data sources, so it’s our responsibility to tease out what is truly innovative and ascertain its value to the investment process.
Aminah: How have you seen the rise in amateur retail trading affect the industry? Is your organisation keeping monitoring data it previously wasn’t? e.g forums/reddit etc?
George: We do monitor overall trends of retail investor and identify specific stocks where they are particularly active. I sometimes joke that the best source of information in this space is my teenage son. But I’m not really joking because we try to steer clear of investors flocking to a stock just because they think someone will come along tomorrow and buy it for a higher price. From our perspective, fundamentals drive long-term prices, but it can take some time for this to occur when something becomes a “fad” stock.
We avoid chasing fads that retail investors can often be drawn to, such as meme stocks or crypto in the short term. Our priority is assessing and finding the advantages for our clients within the durable waves of change that we are experiencing, be it top-down trends or bottom-up. While we’re cognizant of market trends our priority is to disaggregate between a short-term fad and a long-term market driver. As quant investment managers, our goal is to filter out information from the noise. Information should be incorporated into our investment process while noise should be handled with smart risk management. The challenge with markets is that it’s possible for large groups of investors - like retail investors - to create a self-fulfilling result for an unpredictable period of time. So, while we may have periods when market participants can drive prices away from long-term fundamentals, it’s very difficult to time when the temporary move will revert to the norm. And while things may differ for a “short” period (whatever short may mean), long-term investment prospects will continue to be determined by fundamentals.
Aminah: A portion of the industry still feel that advanced ML techniques such as Reinforcement Learning and Deep Learning cannot be applied to financial data effectively – do you agree? What are the main challenges in preventing this from happening? Can you give some concrete examples where you’ve seen this work successfully?
George: There is no doubt that machine learning has been effective in many areas of computer science and engineering. However, the challenges faced by financial markets include the very high noise-to-signal ratio along with the many applications of machine learning and practitioners that operate at different horizons. And while most practitioners consider predicting future returns as the “holy grail” of ML, I have seen it be very effective in data cleaning and error identification, which is often a thankless job. In addition, the language processing aspects of ML have been very effective in both extracting numerical data as well as measuring sentiment associated with various documents. Personally, I believe that NLP will ultimately become the most valuable type of ML used in financial markets due to the huge amount of written analysis that is produced by the industry, as well as the overall complexity of these documents.
With regard to deep learning, these models have a significantly larger number of parameters that need to be estimated (calibrated), and thus the amount of data needed to estimate such a model substantially increases. For instance, if you’re looking to predict future prices over the next few seconds, then there might be enough independent data available to make this possible. But at longer horizons, such as over several months, you quickly run out of data. And so, the challenge in these scenarios is that amid different regimes the relationship between inputs and outputs is not stable. In the world of science, this never happens. There is never a bad year for gravity, but this can easily happen for a given factor in financial markets.
For Professional Investors only. All investments involve risk, including the possible loss of capital.
The content and materials presented here are for informational and educational purposes only and should not be construed as investment advice or an offer or solicitation in respect of any products or services to any persons who are prohibited from receiving such information under the laws applicable to their place of citizenship, domicile or residence. PGIM Quantitative Solutions LLC (PGIM Quantitative Solutions or PGIM Quant), formerly known as QMA LLC, is an SEC-registered investment adviser and a wholly-owned subsidiary of PGIM, Inc. (PGIM), the principal asset management business of Prudential Financial, Inc. (PFI) of the United States of America. Registration with the SEC does not imply a certain level of skill or training. PFI of the United States is not affiliated in any manner with Prudential plc incorporated in the United Kingdom or with Prudential Assurance Company, a subsidiary of M&G plc, incorporated in the United Kingdom.
Don't miss George Patterson at Quant Strats Europe 2022, LIVE and in-peron at the Park Plaza Victoria, London with 40+ top experts and 200+ attendees, and learn more about the quantitative data industry. Join George Patterson at his exclusive Keynote fireside chat/presentation as he closes our event on the 13th of October 2022.
Download your free copy of the agenda by clicking here.