Interview: David Murdock, CFA, VP of Product Management for Data Services, Visible Alpha
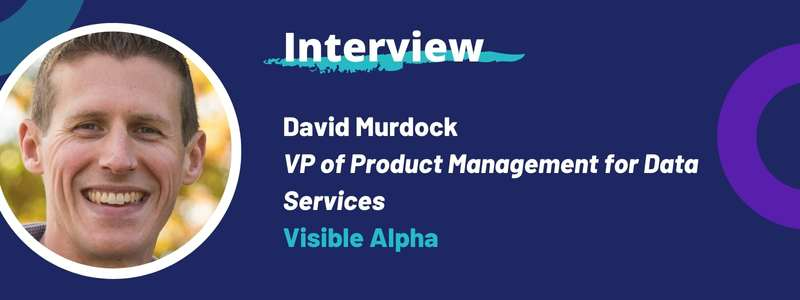
Please give us a little introduction on your current role and what you do
My name is David Murdock, CFA, and I’m VP of Product Management for Data Services at Visible Alpha. In my role, I’m responsible for delivering Visible Alpha’s unique forecast and historical company data through various distribution channels, such as APIs, Feeds, and Cloud. These solutions are designed to give quantitative analysts, data scientists, application developers and other programmatic users the content they need in the platform of their choice.
What do you consider your biggest professional achievement to date?
I spent the bulk of my career (20 years) at FactSet helping build out their Content and Technology Solutions (CTS) business. Through a variety of sales and product leadership roles, I played a key part in taking what was a small side business and growing it into one of the three main business units at the firm. Visible Alpha offered me the opportunity to do that again, and I’m leveraging that experience to try and achieve something similar here.
What has been your /your firm’s top 3 priorities for the coming year? What are you hearing from your clients as their main priorities?
My top three priorities both this year and next are as follows:
1) Data Discovery and Usability: Our data set is large and complex, requiring the user time to get up to speed. How do we make that onboarding process simpler? What can we do in terms of curation, flags, and tagging to make our data more discoverable?
2) Data Expansion: We are always looking for ways to create new data sets that will solve a customer's problem or improve their workflow. For example, this year we released valuation metrics on our platform, which was a highly requested content set. We continually experiment with new ideas and collaborate with clients to understand what new data products we should invest in.
3) Data Delivery: Our goal is to meet the customer in the environment of their choice. How do we get our data seamlessly integrated into the client’s systems? Right now we offer data via our interactive web application, Excel add-in, APIs, feeds, and Snowflake. There is a big push in the industry for cloud delivery, and we are evaluating which platforms beyond Snowflake we want to work with to help reach more customers.
These initiatives are all designed to help what we are hearing from our clients, who are trying to sift through the noise and find data sets where they can find value quickly. They spend a disproportionate amount of time finding, cleaning, and aggregating data into a usable form for their research process. Our goal is to streamline that so they can spend more time analyzing the data and improving their investment models.
What do you think are the biggest challenges facing data scientists/AI experts/quantitative practitioners for 2022 and beyond?
One of the biggest challenges I see facing quantitative data users is the decentralization and proliferation of content. The sheer amount of options is compounded with each vendor having proprietary delivery platforms and commercial terms, making it difficult to test all the data sets that may add value to your process or even just keeping ongoing data pipelines in good working order. These teams have a finite and often static amount of resources that simply can’t keep up with the continually expanding data offerings in the financial data space.
Alternative data is still considered a source of alpha for many – what roadblocks do firms tend to come across in sourcing, cleaning, and using this data? How do you view the alt data market at present and where is it heading? How can we streamline this process and is that possible?
Firms continue to be overwhelmed with the amount of data out there in the marketplace. I see two common challenges:
1. A lack of resources to get these sources of data into a usable form at a scale
2. A lack of knowledge around how to get utility out of the data
I believe the alt data market will continue growing rapidly, which will only compound the problems. To streamline this, more industry standards need to be created and adopted. For example, we don’t have a universal symbology to identify companies and financial instruments. Clients spend time with each data set just trying to ensure they are accurately matching the securities in their database with that of the vendor. In addition, in order to set up a pipeline to receive data, the client typically has to learn a proprietary API or build an ETL for the flat files that a vendor provides. We tackled this to some degree with data sharing via Snowflake, but can we get the industry to consolidate around 1-2 data marketplaces? Until there is a common platform and standards that data vendors conform to clients will continue to have bespoke setups for each vendor they work with.