Interview: Daniele Grassi, Co-founder and CEO, Axyon AI
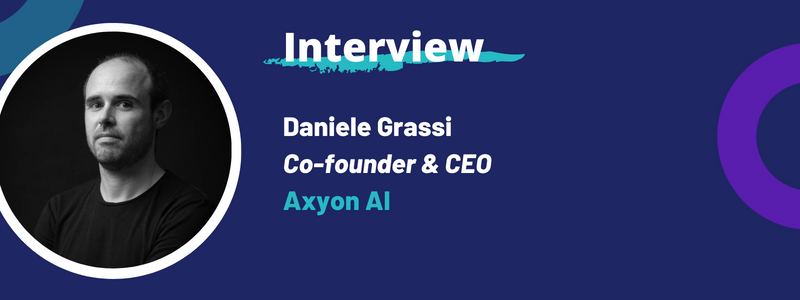
Aminah (Content Lead at Quant Strats): Hi Daniele, we are delighted that Axyon AI has decided to be a sponsor for Quant Strats Europe. We would love to know more about you. Could you give us a little introduction to your current role and what you do?
Daniele: Thank you. It's great to have a chance to speak with you. My name is Daniele Grassi, I am co-founder and CEO of Axyon AI. At Axyon AI we develop AI-powered investment strategies that help asset managers perform better in the market.
Don't miss new reports! Sign up for Quant Strats Newsletter
Aminah: What do you consider your biggest professional achievement to date?
Daniele: One of my greatest achievements is having been able to grow Axyon AI in the AI for investment management without compromising scientific rigour and professionalism. As a result of complex dynamics, the signal-to-noise ratio in financial markets is extremely low; this means that stormy weather is always surrounding you, so it is vital to take a long view of the future and focus on the soundness of your processes rather than the short-term results, while at the same time never stop learning from your mistakes. Axyon AI embeds this belief at its core. The constant research of excellence is what characterizes our team, and has helped to create this kind of culture is something that makes me extremely proud.
Aminah: That's great. It sounds like your culture has been a driving force behind the success of Axyon AI. And, What have been your /your firm's top 3 priorities for the coming year? What are you hearing from your clients as their main priorities?
Daniele: In 2023 we will focus on what we call "delivery"; having reached a good product/market fit in the last year, we are now working to simplify the adoption of our technology into investment products. In addition, we will be greatly expanding our assets coverage and increasing the amount of data that informs our AI models. Lastly, we will leverage our latest fundraising to improve our dissemination and lead generation capacity.
Aminah: What do you think are the biggest challenges facing data scientists/AI experts/ quantitative practitioners for 2022 and beyond?
Daniele: The biggest challenge is data quality. Although this has been discussed for a long time, most data providers haven't yet reached the level of data quality and delivery reliability that is a necessity when it comes to employing machine learning-powered algorithms. For example, point-in-time data is hard to come by for many of the time series that are commonly used; when data is not specifically advertised as "point-in-time", it is often very difficult to understand the real backfilling policies that have been used. And unknowingly feeding back-filled data to your machine learning models is something that can easily kill any edge you may have.
Aminah: A portion of the industry still feels that advanced ML techniques such as Reinforcement Learning and Deep Learning cannot be applied to financial data effectively – do you agree, and can you elaborate?
Daniele: Machine learning includes a variety of techniques, each of them with different strengths and constraints when it comes to actually using them. So, it is natural that a few of them do not have a specific fit with financial data. In particular, financial data is time-dependent by nature, and this makes it difficult to use most of the common data handling techniques (such as traditional data splitting methods - that may end up providing extensive information leak from data used for training and data used for validating the AI models). This in turn creates the conditions for overfitting risks, and this may become extremely painful in terms of models' underperformance once live. In addition, I believe that one of the most important overfit risks is constituted by human bias in evaluating results: every experiment you do in machine learning reduces the probability that the results you see on past data will be confirmed once live. This is somehow counterintuitive for humans, and even the smartest data scientists sometimes slip into underestimating this problem. So no, I do not agree, and I believe that it is not a matter of "if" you can effectively apply deep learning (or other advanced machine learning techniques) to financial data. It is a matter of how you do it and how much you are willing to challenge your knowledge and code every day.
Aminah: That's a very insightful answer. It's definitely about the challenge of it. Well, thank you, Daniele, for joining us today. I'm sure our members learnt something important from the interview.
Daniele: Thank you for having me.
Don't miss Axyon AI at the LIVE in-person event at Quant Strats Europe on the 13th of October at the Park Plaza Victoria, London, as we delve further into ML, AI, Reinforcement Learning and Deep Learning. Download your free agenda copy by clicking here.